Do higher scoring students get assigned
higher value-added teachers? Maybe— but more work is needed to really tell.
Early in 2014, Chetty, Friedman and Rockoff published a study based on New York City data that found that
value-added (VA) models which control for the previous years’ test scores can
accurately predict teacher impacts on current year student test scores. Along
the way, the team demonstrated the viability of a quasi-experimental design
that most districts— with student test and teacher assignment data— could use
to validate district VA calculations.
In the past several months, two studies have
replicated the Chetty et. al.’s
methodology and key results in two different samples: a study using data from Los Angeles by Bacher-Hicks, Kane and Staiger and one using data from North Carolina by Jesse Rothstein, who has been academia’s most
vocal critic of value-add methodologies.
Following the specifications and techniques of
the original paper, the two newer papers find little evidence of bias in
teacher VA measures (with the typical controls) and show that there are
differences in teacher VA across students and schools.
All three studies found a significant,
positive relationship between a student’s prior year test score and teacher VA.
In other words, higher-scoring students do get assigned to high VA teachers, on
average, with only some disagreement over what happens to special education
students.
Rothstein (North Carolina) found that minority students (Black or Hispanic)
and schools with higher fractions of minority students have higher VA teachers,
on average. Chetty et al. (New York City)
found no such relationships. Bacher-Hicks
et al. (Los Angeles) found
significantly negative relationships at the student and school levels for
African-American and Hispanic students. In other words, in Los Angeles, African-American or Hispanic students or schools with
higher fractions of these students have teachers with lower VA, on average.
A key benefit of replicating research (which
happens much too rarely in education research) is that in addition to
comparing results in different locales, researchers can identify potential
weaknesses in a study’s design. While Rothstein
replicated the Chetty et al.’s
results using their techniques and specifications, he also went on to
demonstrate two potential flaws that should be the subject of future work: (a)
the results change depending on how one makes up for missing classroom or
teacher data; and (b) the “random” teacher moves— from classroom to
classroom or school to school— required for the quasi-experiment’s validity are
not so random after all. Taking these into account, he finds significant bias
in teacher value-added calculations: for some teachers, the models predict
better performance, on average, than they should, while for other teachers, the
models go the other way.
What’s the bottom line of these studies? There
seems to be clear evidence that in New
York City, Los Angeles and North Carolina, higher-scoring students
are assigned to higher VA teachers, on average. There is significant variation
across the three jurisdictions in the relationships between a student’s special
education or minority status and average teacher VA scores. Finally, Rothstein raises significant questions
about validity with respect to resolving missing data and the independence of
teacher turnover. All of these require further hypothesis testing— and more
replication studies.
More like this
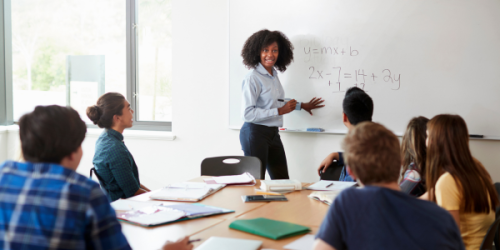
Districts are facing hard choices: How can teacher evaluation help?
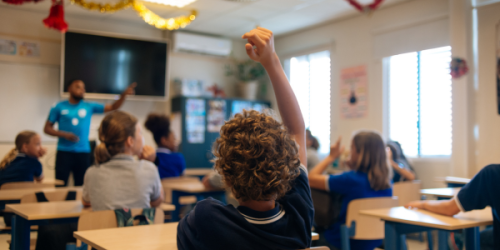
Rural teacher evaluation system shows promising results for students struggling in math
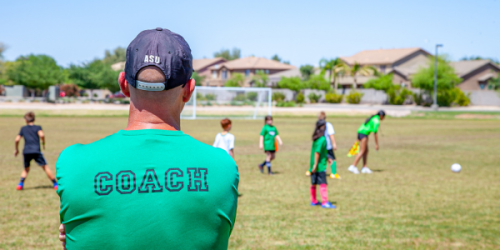
Put me in, coach! How practice plus coaching helps aspiring teachers win
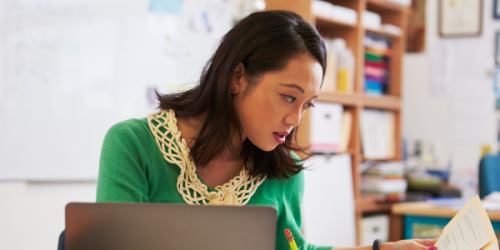